Application of Machine Leanring in BioScience Research
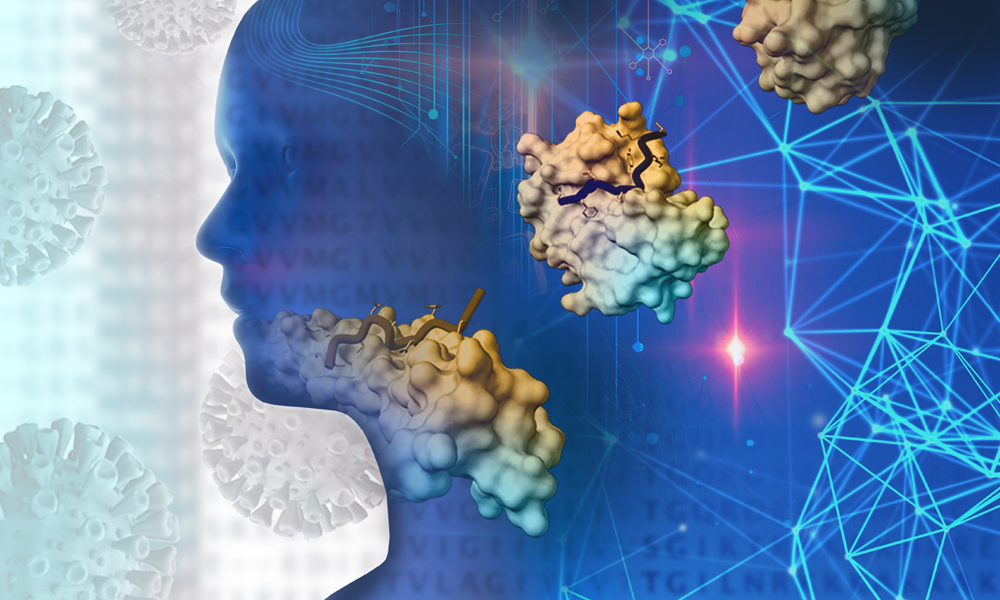
Machine learning (ML) has made significant contributions to bioscience research, revolutionizing the way biological data is analyzed, interpreted, and utilized. Here are several applications of machine learning in bioscience research along with examples:
- Drug Discovery and Development:
- ML algorithms are used to predict the bioactivity and toxicity of drug compounds, accelerating the drug discovery process.
- Example: Atomwise uses deep learning to screen millions of chemical compounds for potential drug candidates, leading to the discovery of new treatments for diseases such as Ebola and multiple sclerosis.
- Genomics and Personalized Medicine:
- ML techniques analyze genomic data to identify genetic variations associated with diseases, predict patient outcomes, and personalize treatment plans.
- Example: DeepVariant, developed by Google, uses deep learning to accurately identify genetic variations from DNA sequencing data, aiding in the diagnosis of genetic disorders and cancer.
- Proteomics and Protein Structure Prediction:
- ML models predict protein structures, functions, and interactions, facilitating protein engineering and drug target identification.
- Example: AlphaFold, developed by DeepMind, utilizes deep learning to predict protein folding structures with high accuracy, advancing our understanding of protein biology and drug design.
- Disease Diagnosis and Biomarker Discovery:
- ML algorithms analyze clinical and biological data to diagnose diseases, identify biomarkers, and stratify patient populations.
- Example: IBM Watson for Oncology uses ML to analyze patient data and medical literature to assist oncologists in diagnosing and treating cancer, improving patient outcomes and treatment decisions.
- Neuroscience and Brain Imaging Analysis:
- ML techniques process neuroimaging data to study brain function, identify biomarkers of neurological disorders, and develop diagnostic tools.
- Example: Convolutional neural networks (CNNs) analyze functional MRI (fMRI) data to map brain activity patterns and identify neural networks associated with cognitive functions and mental health disorders.
- Biomedical Imaging and Pathology:
- ML algorithms analyze medical images such as X-rays, CT scans, and histopathology slides to detect abnormalities, assist in diagnosis, and predict patient outcomes.
- Example: Google’s DeepMind Health uses deep learning to analyze retinal images and identify signs of diabetic retinopathy, enabling early detection and intervention to prevent vision loss.
- Drug Repurposing and Side Effect Prediction:
- ML models analyze drug-gene interactions, biological pathways, and clinical data to repurpose existing drugs for new indications and predict potential side effects.
- Example: BenevolentAI applies ML algorithms to identify novel uses for existing drugs, such as repurposing antidepressants for treating neurodegenerative diseases like Alzheimer’s.